Guides Engineering Best Practices AI & LLMs
8 Best Practices to Understand and Build Generative AI Applications Effectively
Big tech companies and startups alike are pouring resources into AI research and development, hoping to harness its potential for boosting efficiency, improving decision-making, and unlocking new revenue streams. But the hype often overtakes reality, leading to unrealistic expectations—and some misconceptions—about what AI can actually do. This situation is a bit like the early days of crypto: both AI and crypto started as disruptive technologies—but unlike cryptocurrency, which faced fundamental economic concerns and a lack of real-world use cases people could connect with, AI has seen broader adoption and investment because of its real-world applications and success stories, especially in natural language processing (like us with Query Assistant). These successful implementations have shown AI’s practical benefits and potential for innovation. Companies that have successfully integrated GenAI into their operations are reaping the rewards in terms of competitive advantage and operational efficiency, but those who have done so often limited their scope and focused on specific and narrow problems to solve. In this guide, we’ll help you get there by sharing eight best practices to help your organization understand and build GenAI applications more effectively.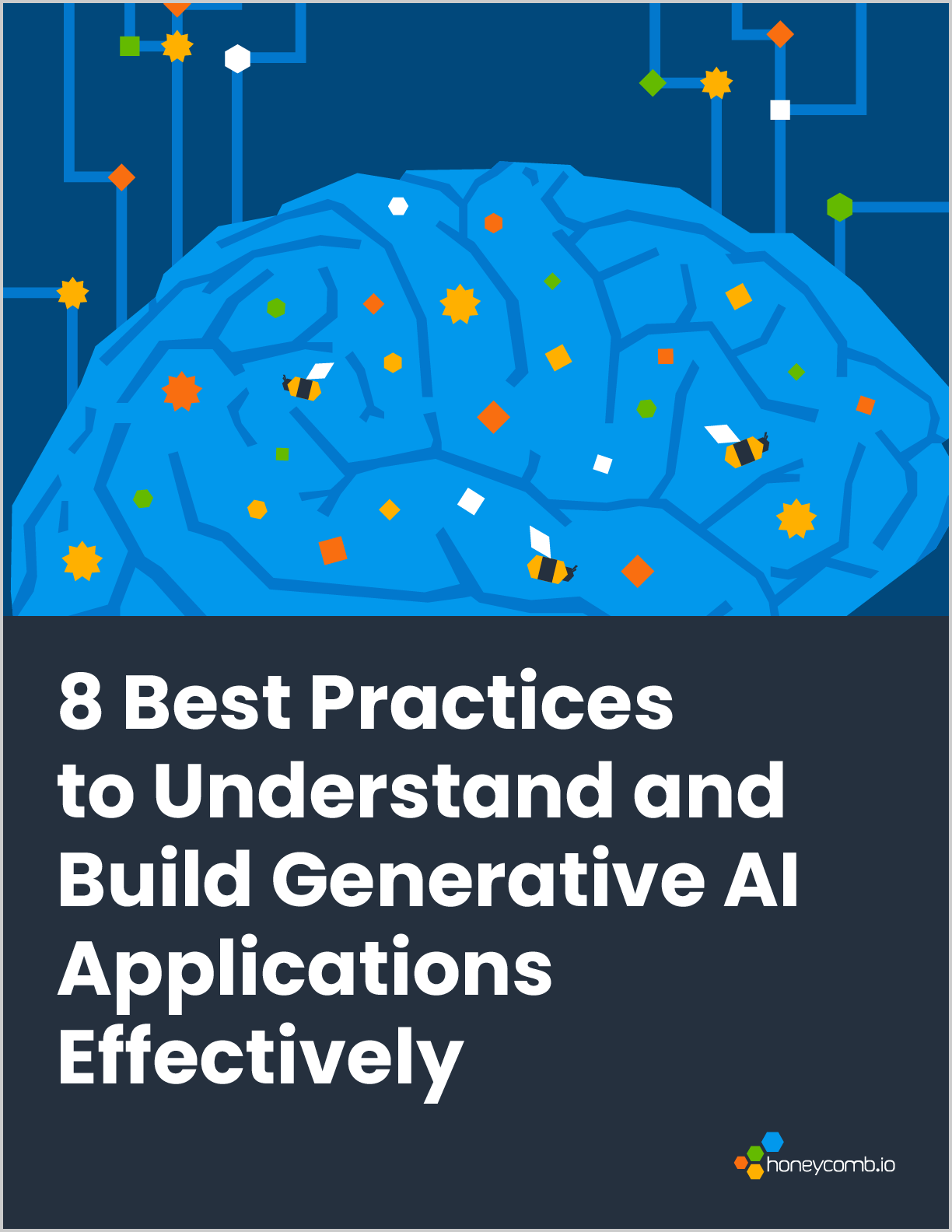